Eleanor Tursman is an Emerging Technologies Researcher at The Aspen Institute, where they work at the intersection of novel technologies, public education, and public policy. They were a Siegel Research Fellow from 2022-24.
Prior to joining The Aspen Institute, Eleanor served as a TechCongress fellow in the office of Representative Trahan (D-MA-03), providing technical and scientific support for topics including health technology, educational technology, artificial intelligence, algorithmic bias, and data privacy. They also worked as a computer vision researcher at Brown University, with a specialty in 3D imagery and the human face. Their research centered on finding new ways to approach deepfake detection, with a focus on long-term robustness as fake video becomes harder to visually identify. Eleanor has an M.S. in computer science from Brown University and a B.A. in physics with honors from Grinnell College.
Below is a summary of Eleanor’s conversation with Siegel Emerging Technology Advisor, Eryk Salvaggio.
Eryk: When do you remember first being curious about a technology?
Eleanor: The first piece of tech that I really got into were oscilloscopes in my introductory physics course in college.
Eryk: That’s those flickery green screens that show you voltage spikes, right? A lot of early computer and electronic art used those, too.
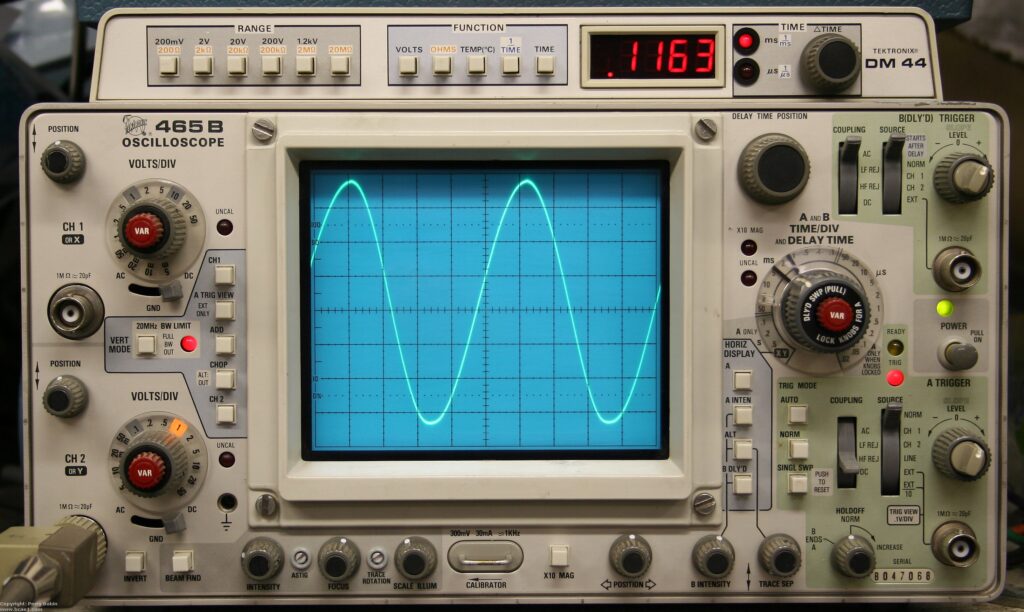
Eleanor: Yes! It was exciting to use a device to visualize a thing, in this case voltage, that I can’t see with the naked eye. Since that point, I’ve been interested in signal processing and visualization tools like cameras. Cameras transform physical information, light information, into a signal that a computer can process. Thinking about how you process that information was what made me interested in computer vision. I went to grad school for computer science, focusing mainly on 3D imaging – taking multiple camera views or video views of a scene, and then inferring the 3D properties of the scene. It combined my interests in physics, computer visualization, and signal processing.
I was interested in the human face, because unlike research on 3D models of architecture, chairs, or rigid objects, the human face is not rigid. It can transform in interesting ways and that’s a hard thing to process. If you pick up a cup, you have a rigid object. You can rotate it and translate it in space, but can’t actually make it deform like putty or clay. But the face can do all kinds of funny things: you can scrunch it up or make it really big. It’s a non-trivial problem to process that complicated geometry, as it’s changing through time and space, into a way that’s useful for a computer.
But I was frustrated with what I felt was a dominant academic push towards generating better synthetic faces, without robust conversation about the ethics of that. NeurIPS, one of the big machine learning conferences, didn’t even have its ethics requirement when I started grad school (Note: they do now). The field felt different at the time. I wanted to do something with faces that didn’t feel ethically dubious.
My computer vision research centered on synthetic image detection. How do you tell if something’s been manipulated? There’s always going to be an arms race: better generators, better detectors. We postulated that, in a very constrained scenario, different camera views of the same event can show you what the face geometry should be at every point in time based on all of these different perspectives. So then if someone tries to manipulate a video of this event, changing the way the speaker’s mouth is opening or closing, you can check the suspect video’s geometry against this consensus-based geometry for any inconsistencies.
I also felt frustrated working in academia because of the slowness of response, and feeling like what I was doing didn’t really have an impact. So that’s when I pivoted into policy. I did a TechCongress Fellowship, which allowed me to lend my expertise to members of Congress.
Eryk: How did you find working with AI ethics in a policy environment?
Eleanor: I don’t think of myself as an “AI and ethics” person. I don’t have an ethics background. I think of myself more as an interdisciplinary connecting piece, a person who can talk to scientists, policymakers, lawyers, community advocates, and members of civil society, and make sure that they’re meeting and understanding one another.
In my work at Aspen, I think of myself as a person trying to make full ecosystem adjustments, as opposed to only making adjustments in academia or only making adjustments in public policy. That work is important, but I’m in a unique position to bring together experts and leaders from different disciplines and parts of society to chart a path forward.
I am largely working on AI policy right now. Something that B [Cavello] and I like in the emerging tech team at Aspen Digital has been to focus on positive potentials of AI. So not only the important work of reducing harms, but the work of figuring out what kind of futures people actually want to be building towards with this technology? What does the public want to see from AI as opposed to what a select few in Silicon Valley think the future of AI should be?
Eryk: I relate to that, and I think that connector role is undervalued –
Eleanor: I think it is. It’s definitely underfunded, at least within the academic context. Interdisciplinary work in the sciences is not structurally motivated, based on the way you get grants and the way you work. So in practice, you aren’t incentivized to work across disciplines, which is silly because, for example, the computer vision research I was doing is related to cognitive science and neuroscience. There’s a clear intersection. It produces better work overall when research is more interdisciplinary.
Eryk: Your work with the Reporting on AI Hall of Fame was organized to celebrate great writing about AI. What inspired that project?
Eleanor: When I first started at Aspen, we were developing AI primers to help people understand a technology that many people were just finding out about for the first time. We were looking for accessible news articles about different subtopics, especially in generative AI. AI is, in my opinion, a purposefully confusing term that captures different types of technology under its umbrella. So it’s hard to write about, especially when most of the educational resources out there are published by companies, which have an incentive to present their technology in a certain way. Reporters covering technology are often generalists, which means that they cover a range of tech-flavored things – product launches, email security breaches, tech mergers, and now AI. They may just need a quote and have three hours to do it. The primers aim to help them incorporate many more perspectives.
So we were looking for examples of good writing about AI that we could refer people to, but we found a wide range in quality. We realized there might be an opportunity to more explicitly help people talk better about AI.
While doing a hall of shame would have been fun, it’s not fun or helpful for the people you’re shaming. Not only that, but if you celebrate the good work people are doing, they will help you get the word out about it. We have a positive frame for tech policy. We want to encourage a shift in thinking: can we highlight this good writing in some way? Good writing is not getting enough recognition, and it is quite hard to do well. That was the impetus for the Hall of Fame.
Eryk: What did you learn in the process? What did people do when they did AI writing well?
Eleanor: We learned that good writing comes from a lot of different places, not just the mainstream media. For example, student newspapers and the FTC business blog. In reading lots of submissions we also developed a rubric of things we value in writing well about AI tools.
For example, one rubric component focuses on NOT personifying AI tools. It’s better to say, “The manager used this AI tool to fire people” instead of “The AI fired 100 people.” Blaming AI obfuscates the actual actor, which is the manager in this case, and shields them from accountability.
Eryk: This led to these AI media literacy lesson plans from Aspen Digital. How do you define AI literacy?
Eleanor: For me, AI literacy is being able to critically interpret information about AI across different types of media. I don’t think that it’s fundamentally different from normal media literacy, only more complex. The general public doesn’t necessarily understand what AI tools can be used to do, what types of tools are out there – or, really, what actually is and is not AI. Combined with an industry that has an incentive to overstate capabilities, it creates a difficult environment for literacy around this tech stack.
It’s further complicated by the fact that we as people have a bias (called “automation bias”) to believe that an output from a computer is more reliable than an output from a person. It makes sense in some ways. I trust a calculator to solve an arithmetic problem much more than a person doing it in their head. Lots of folks interact with AI like it’s a calculator, like it can produce a hard coded answer every time when that’s just not the case.
Our media literacy plans are for teachers working with late high school students, who are at the forefront of using these tools. We tried to make it very user-friendly through user interviews and we released it as an open education resource under a very permissive Creative Commons license to encourage people to take it and remix it and use different parts of it however they want.
Eryk: Broadly speaking, what do you think are the challenges and obstacles you face when it comes to public literacy around AI and, separately, generative AI?
Eleanor: A key issue in AI literacy is what the systems are actually capable of being used to do. There’s a lot of stuff out there that is technically machine learning, but that people don’t necessarily think of as machine learning. The autofocus in this camera we’re using for this video call has a facial recognition component. The transcript that will come out of this conversation will include the line “AI-generated content may be inaccurate or misleading. Always check for accuracy.” And if I say that, a reader might think I mean this whole conversation was generated using ChatGPT, when I actually mean that the audio is being transcribed using an AI model trained for speech-to-text applications.
Eryk: Though perhaps it’s ok that we don’t call it all “AI” so we’re not constantly mystified by technology. We can think and talk much more clearly when we define what we’re talking about – like autofocus or voice transcription software.
Eleanor: Exactly. Another issue is personification. The systems are presented as personified chatbots (by companies), which works in their interest and fulfills our human desire to personify things. So a teacher might assume that they could ask, “Hey, ChatGPT, is this essay plagiarized?” But the system itself is fundamentally just a text generator. There is no factual processing; it’s just going to generate nice sounding text about a topic that you give it. The basic literacy I think we need is that there are fundamental limitations for any of these systems.
And another issue is that the public cannot always tell when the impacts they are feeling are due to AI use. I think it’s very difficult for the average person to think about how technology impacts their day-to-day life. It’s not until there’s a problem – like identity theft or denied coverage due to an automated decision – that people realize the risks.
For example, when I go to the airport, everyone uses the facial recognition check-in. Why wouldn’t they, when it’s presented as the default option? The narrative around the harms of facial recognition, especially when it comes to poor performance recognizing people of color, is well established in the public consciousness. But can the typical traveler really conceive of that information potentially being sold to a data broker and then impacting them five years from now because the data was never deleted from a database? (TSA does have some more strict data storage guidelines, for what it is worth.) The link between cause and effect is so removed. That makes things complicated legally too.
Eryk: Right! Wherever people stand on the training data conversation, one of the recent complications is that the people who gathered the data were not the people who turned it into a commercial model and therefore they are not liable. The pipeline of data is used to obfuscate the relationship between uploading an image and having it show up in facial recognition software. But on the other hand, if you want to do research, of course you get data where you can – how else would we have, say, hate speech research into a social media platform, if you can’t look at and analyze what’s being said? But there is an abstraction and obfuscation between research and commercial uses.
Eleanor: Making your own data sets is really expensive. So grad students that are building these data sets for their projects are incentivized to scrape places like YouTube, Flickr, and Wikipedia. I wish there was a better incentive structure for dataset creation and management and stewardship because the current system is not good for the public.
Eryk: What do you think are the challenges in AI, what needs to change, and what resources are needed to make that change?
Eleanor: Media literacy, especially K-12, is really important. I don’t think everyone needs to know what AI is under the hood or how it works. You don’t need to know how your car works to drive your car!
However, you should know the warning signs that your car is breaking down. You need to be in an ecosystem where you trust that there is an engineer that can fix your car. You trust the gas that comes out of pumps that goes into your car. You need to know how to operate the car safely to get from point A to point B.
So even if you don’t know the nitty gritty, you do need to know how to use it and what you can reasonably expect from it. That would be the dream for AI literacy.
Eryk: I agree with your point, but isn’t part of the problem that you kind of do need to know how the AI works in order to counteract that? Right now, it’s as if the gas station is telling you to drink the gas. So without that trust in the ecosystem, how do you verify claims about the tech for yourself without learning how to build your own engine?
Eleanor: Well, for example, you don’t need to understand the transformer architecture to talk about what an LLM is. I think that you’re right, that there is one level more than just “this is a tool and these are its limits,” especially because those limitations will change over time.
Eryk: Yeah, I agree. I think of it as studying chemistry to figure out when you need an oil change. Chemistry is involved in that process, but it’s not where you need to be looking. It’s not the appropriate scale for the problem. In similar ways, much of what we call AI literacy is about finding that proper lens for the problems they might create.
Eleanor: Yeah, you just need to know what signs indicate that something is going wrong. What is the sign that your car is about to potentially overheat? What sensor do you look at? What do you smell? How do you feel the car move? AI literacy can translate that into the AI context. I think that would be really powerful.
In terms of resources, I am inspired by community pushes for education – stuff at your local library, making free, accessible tools for people to play with, and community-focused resources. I think education would go a long way, which leads to trends that are giving me hope.
For the past year or so, I’ve been participating in and watching people build things specifically around public AI. Public AI means AI systems that are publicly accessible, publicly accountable, and sustainably public. There are a lot of folks all over the world that are working on whole-of-ecosystem solutions to this problem of AI systems, data, and compute becoming fully enclosed by only a handful of companies for the global market. There is no public accountability for those enclosed systems.
There’s also a disconnect between private industry and what the public might want from these tools. Unfortunately, people are not structurally empowered to say, “I want this thing to exist in the world and I’m going to build it.” One solution might be that we approach AI from an infrastructure perspective. Making that a public utility, making data and access to data and access to compute something that the public can access for free or like at low cost, giving alternatives to private AI options, feels like a way to shift the entire ecosystem in a positive direction.
What could my local library be doing with AI? I don’t know! But that’s the point. We are missing out on so many different people’s perspectives with the way AI is currently being built. Wouldn’t it be cool if public institutions like libraries were resourced to help the public navigate this new opportunity to define what success looks like on their own terms? Pushing for this kind of vision of the future would shift the AI ecosystem in a way that is more aligned with what people might actually want technology to do for them in the future. I think that’s pretty cool.